Digital App May Significantly Boost Ability to Detect Autism at ‘Well-Child’ Pediatric Visit
Digital App May Significantly Boost Ability to Detect Autism at ‘Well-Child’ Pediatric Visit
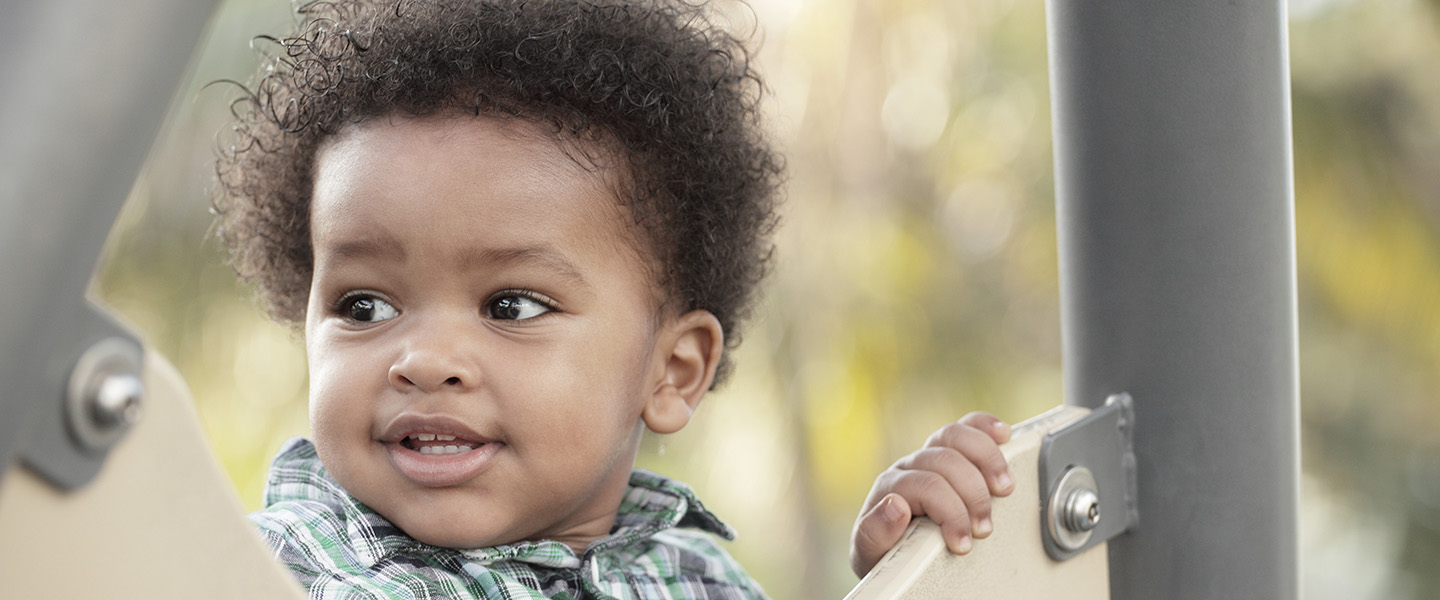
Researchers have developed an app delivered on digital tablet devices that significantly improves upon currently existing tools to screen toddlers for autism spectrum disorder (ASD). Early detection of ASD is widely regarded as critical, making it more likely a child will have prompt access to therapeutic interventions that can improve outcomes.
The new app, called SenseToKnow, was deployed in a multi-clinic setting. In an initial test reported in the journal Nature Medicine, it was administered in 475 cases during a routine “well-child visit,” typically made when a child is 18-24 months of age.
Often, pediatricians give parents a questionnaire at the well-child visit called the Modified Checklist for Autism in Toddlers (M-CHAT). This tool has been shown to have higher accuracy in research settings than in the real world, and especially in cases involving girls and children of color. Another important screening tool uses eye-tracking technology to measure children’s attentional preferences for social vs. non-social stimuli. Autism is characterized by reduced spontaneous visual attention to social stimuli, and while machine-learning analysis of eye-tracking data has yielded encouraging results for its ability to distinguish autistic vs. neurotypical children, it does so on the basis of only one of autism’s various behavioral signs, which also include facial expressions, head movements, response to name, and motor behaviors (including restricted or repetitive behaviors).
The SenseToKnow app involves the display on the tablet of brief movies; the child’s behavioral responses are recorded via the tablet’s frontal camera and are designed to elicit a wide range of autism-related behaviors including those just mentioned as well as social attention and eye-blink rate. It was developed by a team led by psychologist Geraldine Dawson, Ph.D., and electrical and computer engineer Guillermo Sapiro, Ph.D., of Duke University. The team that tested the app and analyzed the results included 2015 BBRF Young Investigator Kimberly L. H. Carpenter, Ph.D., and 2001 BBRF Young Investigator Scott N. Compton, Ph.D., both also at Duke.
The data collected by the app are quantified via computer vision analysis (CVA). Machine learning is used to integrate multiple digital data streams into a combined algorithm that classifies each child as either autistic or nonautistic. It also automatically generates metrics reflecting how well the app was administered—the quality of the data; this generates a statistical “confidence level” associated with the diagnostic assessment made in each case. If the app administration falls below a certain level, it can easily be re-administered to boost the confidence metric.
The app does not “determine” if a child does or does not have ASD. Screening tools like the app are designed to predict the likelihood of the presence or absence of a condition, but are not by themselves able to make a positive determination. Expert clinical examination and various diagnostic methodologies are needed to make a “gold-standard” determination in each case.
Various metrics are used to assess screening tests. Two key metrics are the test’s “sensitivity” and its “specificity.” The more sensitive a test is, the fewer the number of false negatives will be. In other words, as sensitivity increases, fewer cases of the condition are missed. The more specific a test is, the greater the likelihood that someone without the condition will be classified as “negative.” A test with high specificity means there will be fewer false positives—in this case, children classified by the app to have autism who, upon additional clinical testing, are found not to have ASD.
SenseToKnow scored very well in terms of both sensitivity and specificity. In the group of 475 toddlers tested (they ranged in age from 17 to 36 months and included 269 boys and 206 girls), 49 were diagnosed with ASD and 98 were diagnosed with developmental delay without autism; 328 of the children were assessed as “neurotypical.” The percentage of children with ASD and developmental delays was much higher than in the general population, a likely reflection of the fact that a significant fraction of the parents who enrolled their children in the trial were already concerned about their developmental health status.
The key question for the study was: how well did the app fare in terms of sensitivity and specificity? Overall, its sensitivity was 88%; its specificity was 81%. By comparison, in a prior test of over 25,000 children, the M-CHAT questionnaire had an excellent sensitivity of 95%, but a specificity of only 39%. This means the parental questionnaire didn’t miss very many cases of ASD, but it also generated a high number of false positives. Receiving notice that one’s child likely has autism is immensely stressful. When this assessment is incorrect, not only are parents going to be emotionally stressed; they are also encouraged to begin having their child evaluated. One thing a highly sensitive and highly specific test does is it reduces unwarranted emotional stress and directs therapeutic resources to children who really need the care.
While the new app has the advantage of making its determination on the basis of entirely objective, computer-analyzed data, and captures features reflecting the variety of autism-related behaviors and early-warning signals, it remains imperfect. As the researchers note, some autistic toddlers will exhibit only a subset of ASD-related features. Similarly, some nonautistic children may exhibit behaviors typically associated with ASD such as displaying higher levels of attention to nonsocial than social stimuli. Interestingly, when results of SenseToKnow assessments were combined with those from the M-CHAT questionnaire, the “positive predictive value” of the result for each child increased substantially.
Thus, one of the team’s conclusions was that combining available screening methods, specifically the new app and M-CHAT, which are both highly accessible at no or negligible cost to average practitioners, would significantly enhance the accuracy of autism screening in the real-world setting of the pediatrician’s office at the time of the “well-child” exam.
Efforts to improve SenseToKnow are under way, as are larger clinical tests that assess broader samples of the population with more typical rates of ASD and developmental delay (ASD is thought to affect 2.5% of all children, 4% of males and 1% of females; developmental delay affects about 17% of children).
The researchers added a cautionary note: accurate use of screening tools requires training and systematic implementation by primary care providers. Also, crucially, for screening to have impact, “a positive screen must then be linked to appropriate referrals and services.”